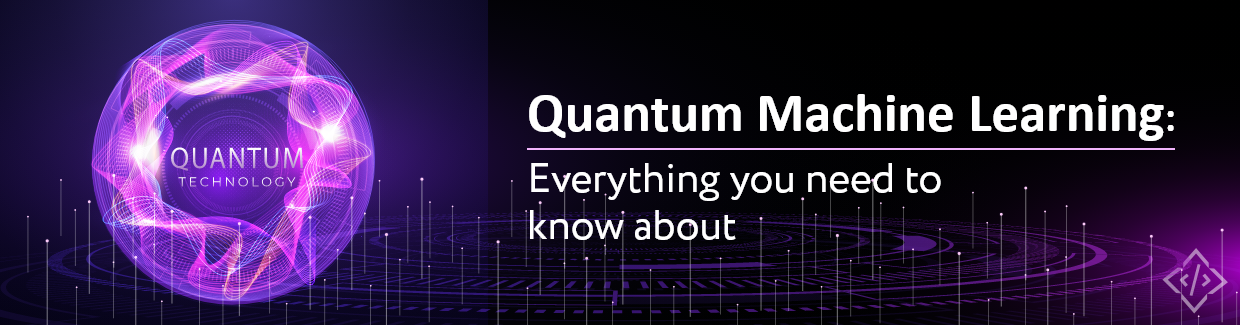
Quantum Machine Learning: Everything You Need To Know About
In the ever-evolving landscape of trending technologies, Artificial Intelligence and Machine Learning, there emerges a cutting-edge intersection that promises to revolutionize the field with Quantum Machine Learning(QML). Quantum Machine Learning (QML) is an emerging field that harnesses the power of quantum computing to enhance traditional machine learning algorithms.
In this comprehensive guide, we delve into the fundamentals of Quantum Machine Learning, its applications, challenges, and the potential it holds for revolutionizing various industries. Let’s start with the basics.
What is Quantum Machine Learning?
Quantum machine learning (QML) is a rapidly evolving field that aims to leverage the unique capabilities of quantum computers to enhance the power of traditional machine learning. At its core, QML leverages the principles of quantum mechanics to enhance the efficiency and effectiveness of machine learning tasks.
Imagine a world where computers can tackle problems that are impossible for even the most powerful classical machines. That's the promise of QML, a burgeoning field that harnesses the bizarre yet powerful principles of quantum mechanics to supercharge traditional machine learning.
Instead of bits (0s and 1s), QML utilizes qubits, which can be 0, 1, or both simultaneously (superposition). This allows for parallel processing of massive datasets and exploring multiple possibilities at once, leading to speed, accuracy, and new possibilities.
Key Components of Quantum Machine Learning
- Quantum Computing Basics
QML requires a fundamental understanding of quantum computing principles, including superposition, entanglement, and quantum gates.
- Quantum Machine Learning Algorithms
These are algorithms specifically designed to run on quantum computers, such as quantum neural networks and quantum support vector machines.
- Hybrid Quantum-Classical Algorithms
As quantum computers are still in the nascent stages of development, hybrid algorithms that combine classical and quantum components are commonly used in QML applications.
Applications of Quantum Machine Learning
- Drug Discovery
QML accelerates the process of drug discovery by simulating molecular interactions and identifying potential candidates for new medications.
- Financial Modeling
QML enables more accurate predictions in financial markets by analyzing vast amounts of data and identifying complex patterns.
- Optimization Problems
QML excels in solving optimization problems, such as route optimization for logistics or portfolio optimization for investment strategies.
- Cryptography
Quantum algorithms can enhance cryptographic protocols, ensuring higher levels of security in data transmission and encryption.
- Natural Language Processing
QML techniques can enhance language processing tasks such as sentiment analysis, language translation, and text summarization, paving the way for more advanced AI-powered communication tools.
- Pattern Recognition
QML enhances pattern recognition tasks in fields like image and speech processing, where complex data patterns can be efficiently analyzed and classified.
Also Read: Artificial Intelligence and Natural Language Processing: A Powerful Combination
Also Read: Conversational AI: The AI That Can Talk Back
Quantum Machine Learning Algorithms
Quantum machine learning algorithms leverage principles from quantum mechanics to enhance traditional machine learning techniques. These algorithms exploit the unique properties of quantum systems, such as superposition and entanglement, to process and analyze data more efficiently than classical computers.
Quantum Approximate Optimization Algorithm (QAOA): Solves combinatorial optimization problems, usually difficult for classical computers.
Quantum Phase Estimation (QPE): Finds the eigenvalues and eigenvectors of quantum states, crucial for various quantum algorithms.
Quantum Boltzmann Machines (QBMs): Neural networks using superposition and entanglement for improved representational power.
Quantum Support Vector Machines (QSVMs): Inspired by classical SVMs, potentially offering advantages in high-dimensional data.
Variational Quantum Eigensolver (VQE): Uses a quantum computer to find the ground state of a molecule that predicts its properties.
Quantum Neural Networks (QNNs): Inspired by classical neural networks that potentially offer advantages for specific tasks.
How Quantum Machine Learning Algorithms Can Perform and Process Data Faster?
QML algorithms have the potential to perform and process data faster due to several key factors:
1. Quantum Parallelism
Quantum computers can perform multiple computations simultaneously through quantum superposition where qubits can represent multiple states simultaneously. This enables quantum algorithms to explore many possible solutions in parallel, potentially leading to faster computation for certain tasks compared to classical algorithms.
2. Quantum Interference
Quantum algorithms exploit interference effects to enhance computation. By carefully designing quantum circuits, interference can be leveraged to amplify the probability of obtaining correct solutions and suppress incorrect ones which leads to more efficient processing of data.
3. Quantum Entanglement
Quantum entanglement allows qubits to be correlated in such a way that the state of one qubit depends on the state of another, even when they are physically separated. This can enable quantum algorithms to perform highly interconnected computations more efficiently than classical algorithms.
4. Quantum Fourier Transform
Quantum algorithms often use quantum versions of classical algorithms, such as the Quantum Fourier Transform, which can efficiently perform tasks like signal processing and data compression. This can lead to faster processing of data in various applications.
5. Optimization Speed-up
Quantum algorithms like the Quantum Approximate Optimization Algorithm (QAOA) and Variational Quantum Eigensolver (VQE) can potentially offer speed-ups for optimization tasks compared to classical optimization algorithms. This is particularly relevant for machine learning tasks that involve optimization, such as training neural networks or solving combinatorial optimization problems.
Overall, quantum machine learning algorithms can process and analyze data faster by harnessing the unique properties of quantum mechanics, such as superposition, interference, and entanglement, to perform computations in parallel and explore solutions more efficiently than classical algorithms.
Challenges and Opportunities
Despite its immense potential, QML is still in its nascent stages, facing several challenges such as qubit decoherence, limited qubit connectivity, and the need for error correction. Overcoming these hurdles requires concerted efforts from researchers and engineers to develop robust quantum hardware, error correction codes, and efficient algorithms tailored for quantum computers.
However, with significant investments from both public and private sectors pouring into quantum research and development, the future looks promising. As quantum technologies continue to mature, the synergy between quantum computing and machine learning will unlock unprecedented opportunities for innovation across industries.
Future Trends in Quantum Machine Learning
As quantum computing technology continues to advance, the future of QML holds immense promise. Key trends of quantum machine learning include:
Improved Quantum Hardware: Advances in quantum hardware, such as error correction techniques and increased qubit coherence times, will significantly enhance the capabilities of quantum computers.
Development of Quantum Software Tools: The emergence of user-friendly software tools and programming languages tailored for quantum computing will democratize access to QML for researchers and practitioners alike.
Integration with Classical Computing: Hybrid quantum-classical approaches will become more prevalent, leveraging the strengths of both classical and quantum computing to tackle real-world problems effectively.
Conclusion
In the dynamic field of artificial intelligence, QML emerges as a transformative paradigm, reshaping problem-solving approaches. QML revitalizes conventional methodologies that push the boundaries of possibility in an ever-expanding future.
QML isn't just about staying ahead of the curve; it's about redefining the fabric of innovation. As we navigate this exciting frontier, let us embrace the challenges, seize the opportunities, and embark on a journey toward a future where quantum meets intelligence in unprecedented ways.
Harness the power of AI and ML for your next project. CodeTrade, a leading AI and ML software development company in India, offers cutting-edge solutions tailored to your specific needs. Discuss your project requirements with their experienced AI/ML developers and stay ahead of the curve!